Book Downloads Hub Reads Ebooks Online eBook Librarys Digital Books Store Download Book Pdfs Bookworm Downloads Free Books Downloads Epub Book Collection Pdf Book Vault Read and Download Books Open Source Book Library Best Book Downloads Jane Clarke Blake Ells Easy Classical Masterworks George C Rable Tom Carpenter Owen R Chandler Special Tactics Terry Ambrose
Do you want to contribute by writing guest posts on this blog?
Please contact us and send us a resume of previous articles that you have written.
A New Paradigm for Primal Dual Interior Point Algorithms: Princeton In Applied

When it comes to solving complex optimization problems, interior point algorithms have emerged as a powerful tool. These algorithms guarantee good theoretical convergence properties and provide efficient solutions for a wide range of applications in various fields.
Among the different variations of interior point algorithms, the primal-dual interior point algorithms have gained significant attention due to their versatility and superior performance. Princeton University, in collaboration with leading experts in the field, has introduced a new paradigm in primal-dual interior point algorithms that promises to revolutionize optimization techniques in applied sciences.
The Power of Primal-Dual Interior Point Algorithms
Primal-dual interior point algorithms are widely used in optimization problems that involve both primal and dual variables. Unlike traditional simplex or active set methods, these algorithms navigate through the interior of the feasible region, hence the name "interior point algorithms."
4.4 out of 5
Language | : | English |
File size | : | 16633 KB |
Text-to-Speech | : | Enabled |
Enhanced typesetting | : | Enabled |
Screen Reader | : | Supported |
Print length | : | 208 pages |
The main advantage of primal-dual algorithms lies in their ability to solve large-scale problems efficiently. This is achieved by exploiting the sparsity of the problem's structure and solving a sequence of well-conditioned, smaller linear systems at each iteration.
Whether it's in linear programming, quadratic programming, or even semidefinite programming, primal-dual interior point algorithms have consistently outperformed competing methods in terms of speed and scalability.
The New Paradigm: Breaking Boundaries
Princeton's new paradigm in primal-dual interior point algorithms aims to address several limitations and challenges faced by existing algorithms. This innovative approach pushes the boundaries of optimization techniques, promising improved efficiency, robustness, and applicability.
One of the key features of this new paradigm is the incorporation of cutting-edge techniques from convex optimization and machine learning. By leveraging recent advancements in these fields, the researchers at Princeton have developed algorithms that can handle non-convex and non-smooth problems with unprecedented accuracy.
Additionally, the new paradigm introduces novel strategies for warm-starting and exploiting problem structure. These techniques allow the algorithms to converge faster and provide high-quality solutions, even for highly ill-conditioned problems.
Applications in Applied Sciences
The impact of Princeton's new paradigm in primal-dual interior point algorithms extends across various applied sciences. From engineering design optimization to finance and machine learning, these algorithms offer a versatile and efficient solution to complex optimization problems.
In engineering design optimization, for example, the new algorithms can handle large-scale problems involving thousands of design variables and constraints. This enables engineers to design optimized structures, ensuring maximum efficiency and reliability.
In finance, where optimization plays a crucial role in portfolio management and risk assessment, the new algorithms enable more accurate and faster solutions. This, in turn, empowers financial institutions to make informed decisions, minimize risks, and maximize returns on investments.
Furthermore, the application of these algorithms in machine learning allows researchers to tackle high-dimensional optimization problems, training complex models with improved convergence rates and generalization capabilities.
The of Princeton's new paradigm for primal-dual interior point algorithms represents a significant advancement in optimization techniques. By combining cutting-edge methodologies from convex optimization and machine learning, these algorithms provide a powerful tool for solving complex problems in various fields.
With their improved efficiency, scalability, and accuracy, the new algorithms offer unparalleled opportunities in engineering design optimization, finance, machine learning, and many other applied sciences.
As researchers and practitioners continue to explore the potential of this new paradigm, we can expect to witness even greater advancements in optimization techniques, further boosting progress in applied sciences.
4.4 out of 5
Language | : | English |
File size | : | 16633 KB |
Text-to-Speech | : | Enabled |
Enhanced typesetting | : | Enabled |
Screen Reader | : | Supported |
Print length | : | 208 pages |
Research on interior-point methods (IPMs) has dominated the field of mathematical programming for the last two decades. Two contrasting approaches in the analysis and implementation of IPMs are the so-called small-update and large-update methods, although, until now, there has been a notorious gap between the theory and practical performance of these two strategies. This book comes close to bridging that gap, presenting a new framework for the theory of primal-dual IPMs based on the notion of the self-regularity of a function.
The authors deal with linear optimization, nonlinear complementarity problems, semidefinite optimization, and second-order conic optimization problems. The framework also covers large classes of linear complementarity problems and convex optimization. The algorithm considered can be interpreted as a path-following method or a potential reduction method. Starting from a primal-dual strictly feasible point, the algorithm chooses a search direction defined by some Newton-type system derived from the self-regular proximity. The iterate is then updated, with the iterates staying in a certain neighborhood of the central path until an approximate solution to the problem is found. By extensively exploring some intriguing properties of self-regular functions, the authors establish that the complexity of large-update IPMs can come arbitrarily close to the best known iteration bounds of IPMs.
Researchers and postgraduate students in all areas of linear and nonlinear optimization will find this book an important and invaluable aid to their work.
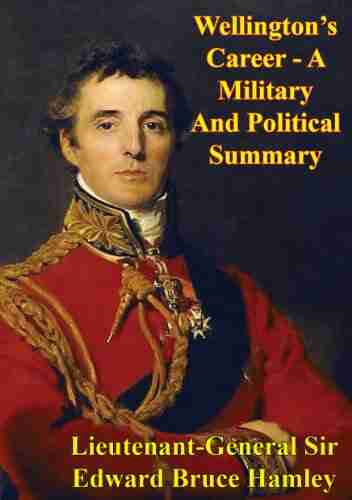

Wellington's Incredible Military and Political Journey: A...
When it comes to military and political...
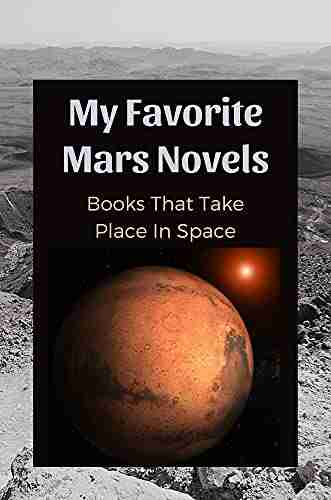

10 Mind-Blowing Events That Take Place In Space
Welcome to the fascinating world of...
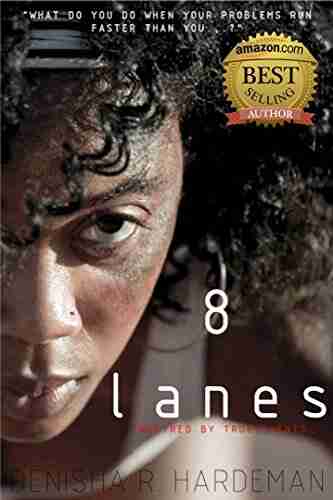

The Astonishing Beauty of Lanes Alexandra Kui: Exploring...
When it comes to capturing the essence of...
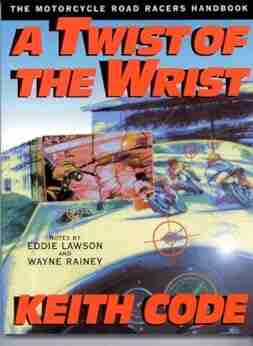

Unlock the Secrets of Riding with a Twist Of The Wrist
Are you a motorcycle...
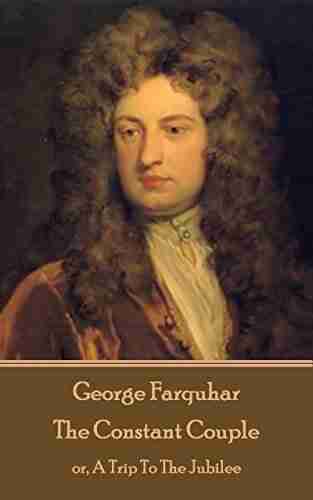

The Ultimate Guide to An Epic Adventure: Our Enchanting...
Are you ready for a truly mesmerizing and...
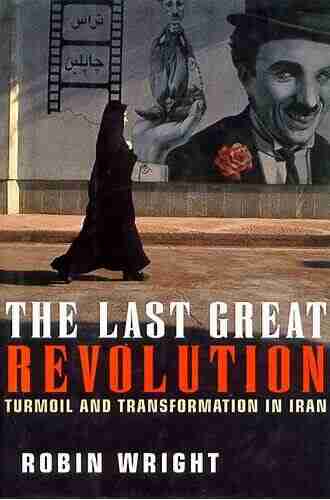

The Last Great Revolution: A Transformation That Shaped...
Throughout history, numerous revolutions have...
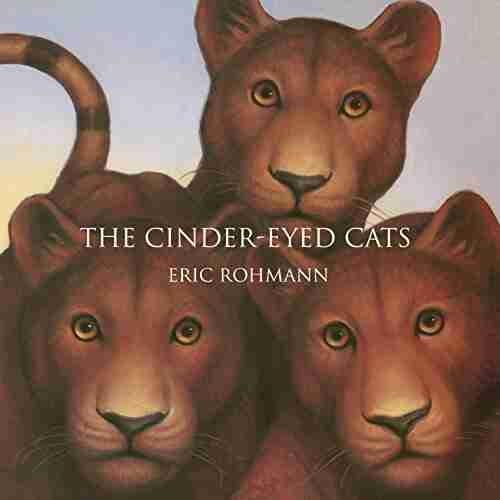

The Cinder Eyed Cats: Uncovering the Mysteries of Eric...
Have you ever come across a book that takes...
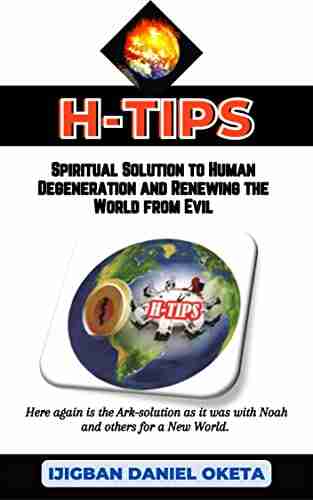

Discover the Ultimate Spiritual Solution to Human...
In today's fast-paced, modern...
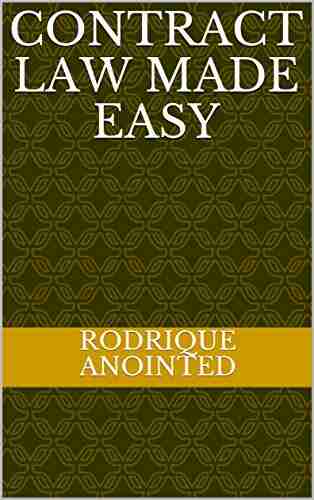

Contract Law Made Easy Vol.: A Comprehensive Guide for...
Are you confused about the intricacies of...
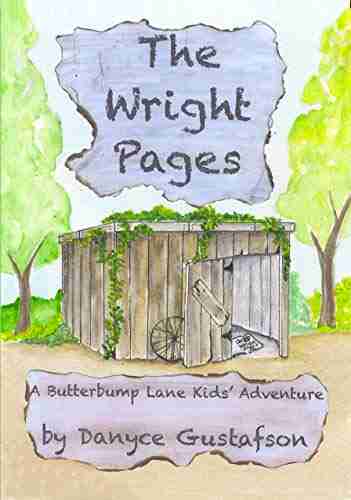

The Wright Pages Butterbump Lane Kids Adventures: An...
In the magical world of...
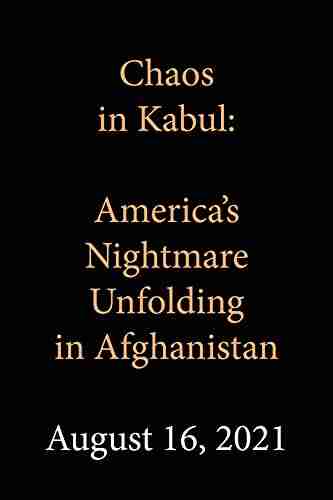

America Nightmare Unfolding In Afghanistan
For more than two decades,...
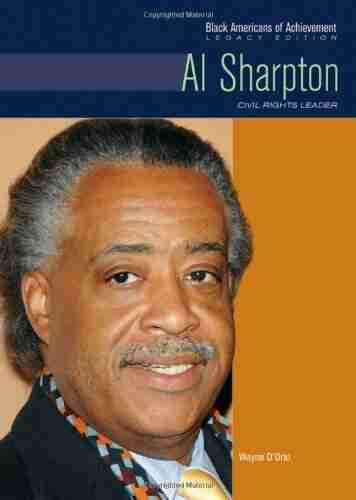

Civil Rights Leader Black Americans Of Achievement
When it comes to the civil...
Light bulbAdvertise smarter! Our strategic ad space ensures maximum exposure. Reserve your spot today!
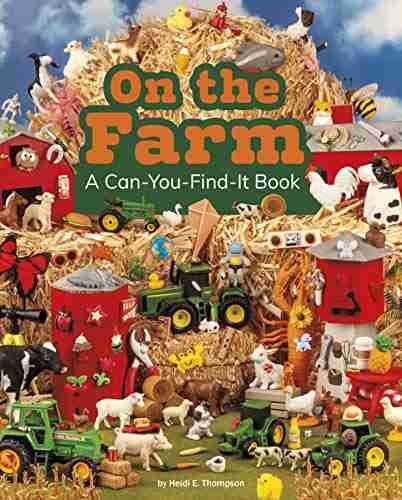

- Anton FosterFollow ·15k
- Graham BlairFollow ·3.3k
- Jeff FosterFollow ·12k
- Brian WestFollow ·15.5k
- Michael CrichtonFollow ·10.4k
- Ernest ClineFollow ·19.6k
- Joseph FosterFollow ·7k
- Darrell PowellFollow ·10.6k