Book Downloads Hub Reads Ebooks Online eBook Librarys Digital Books Store Download Book Pdfs Bookworm Downloads Free Books Downloads Epub Book Collection Pdf Book Vault Read and Download Books Open Source Book Library Best Book Downloads Scott Hildreth Jessica Lage Joseph J Ellis Aldous Huxley Kaplan Test Prep Tom Behrens Richard B Miller Sabrina Taylor
Do you want to contribute by writing guest posts on this blog?
Please contact us and send us a resume of previous articles that you have written.
Unlocking the Power of Bayesian Networks and Decision Graphs in Information Science and Statistics

:
Bayesian networks and decision graphs are powerful tools used in the fields of information science and statistics to analyze complex data, make predictions, and aid in decision-making processes. These graphical models have gained significant attention due to their ability to handle uncertainty, incorporate prior knowledge, and provide a systematic representation of causal relationships.
Understanding Bayesian Networks:
4.3 out of 5
Language | : | English |
File size | : | 3808 KB |
Text-to-Speech | : | Enabled |
Print length | : | 287 pages |
Screen Reader | : | Supported |
Paperback | : | 45 pages |
Item Weight | : | 4.2 ounces |
Dimensions | : | 6 x 0.12 x 9 inches |
Bayesian networks are a type of graphical model that represent statistical relationships among a set of variables through directed acyclic graphs (DAGs). These graphs consist of nodes representing variables and directed edges representing probabilistic dependencies between variables. By modeling probabilistic relationships, Bayesian networks provide a framework for reasoning under uncertainty, making predictions, and conducting what-if analyses.
For example, consider a medical diagnosis scenario. A Bayesian network can represent variables such as symptoms, diseases, and test results, with conditional probabilities assigned to each variable. By observing symptoms and test results, the network can calculate the probability of different diseases, aiding physicians in making accurate diagnoses.
Utilizing Decision Graphs:
Decision graphs, also known as influence diagrams, are another form of graphical model that extends Bayesian networks to include explicit decision-making nodes. These graphs are used to model decision problems that include uncertainties, preferences, and actions, allowing decision-makers to make optimal choices by considering all possible outcomes and their associated utilities.
Decision graphs consist of variables, decision nodes, and utility nodes. Variables represent uncertain factors, decision nodes represent choices that can be made, and utility nodes represent the values assigned to different outcomes. By incorporating probabilities and utilities, decision graphs enable decision-makers to estimate the expected utility of different choices and make informed decisions.
Applications in Information Science:
Bayesian networks and decision graphs find valuable applications in various domains of information science, enabling efficient knowledge discovery, prediction, and decision-making. Some notable application areas include:
- Machine Learning: Bayesian networks are utilized in machine learning tasks such as classification, clustering, and anomaly detection. By learning the probabilistic dependencies between variables, these networks can accurately predict and classify data.
- Information Retrieval: Bayesian networks aid in information retrieval tasks by modeling relationships between documents, queries, and relevance. By considering these relationships, search engines can provide more accurate search results to users.
- Data Mining: Bayesian networks are widely used in data mining to uncover hidden patterns and relationships in large datasets. By utilizing probabilistic inference algorithms, these networks can extract valuable insights from complex data.
Advantages and Limitations:
Bayesian networks and decision graphs offer several advantages in analyzing complex data and making informed decisions. These include:
- Handling Uncertainty: By incorporating probabilistic relationships, these models can effectively handle uncertainty in data and provide probabilistic predictions.
- Prior Knowledge Integration: Bayesian networks allow the incorporation of prior knowledge, improving the accuracy of predictions and decision-making.
- Causal Reasoning: These models provide a systematic way to represent causal relationships between variables, aiding in understanding the underlying mechanisms of complex systems.
- Transparency and Interpretability: The graphical representation of these models provides a visual understanding of relationships and dependencies, making them interpretable even for non-experts.
However, it is important to acknowledge the limitations of Bayesian networks and decision graphs:
- Data Availability and Quality: These models heavily rely on the availability of accurate and reliable data. Insufficient or poor-quality data can lead to inaccurate predictions and decisions.
- Complexity: Building and learning Bayesian networks and decision graphs can be computationally intensive, especially for large and complex datasets.
- Domain Knowledge: Proper domain knowledge is required to define variables, assign probabilities, and specify decision nodes accurately.
:
Bayesian networks and decision graphs have revolutionized the fields of information science and statistics by providing powerful tools to handle uncertainty, make predictions, and aid in decision-making processes. These graphical models have found applications in various domains, enabling efficient knowledge discovery, prediction, and optimal decision-making. While they offer numerous benefits, it is essential to consider their limitations and the need for accurate data and domain knowledge to ensure reliable results. Undoubtedly, Bayesian networks and decision graphs continue to be invaluable assets in the realm of information science and statistics, helping us unravel complex data and make informed choices for a better future.
4.3 out of 5
Language | : | English |
File size | : | 3808 KB |
Text-to-Speech | : | Enabled |
Print length | : | 287 pages |
Screen Reader | : | Supported |
Paperback | : | 45 pages |
Item Weight | : | 4.2 ounces |
Dimensions | : | 6 x 0.12 x 9 inches |
Bayesian networks and decision graphs are formal graphical languages for representation and communication of decision scenarios requiring reasoning under uncertainty. Their strengths are two-sided. It is easy for humans to construct and understand them, and when communicated to a computer, they can easily be compiled. The book emphasizes both the human and the computer side. It gives a thorough to Bayesian networks, decision trees and influence diagrams as well as algorithms and complexity issues.
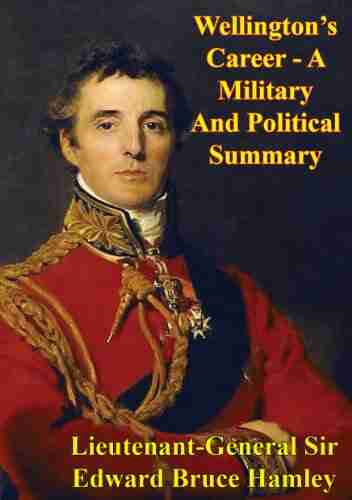

Wellington's Incredible Military and Political Journey: A...
When it comes to military and political...
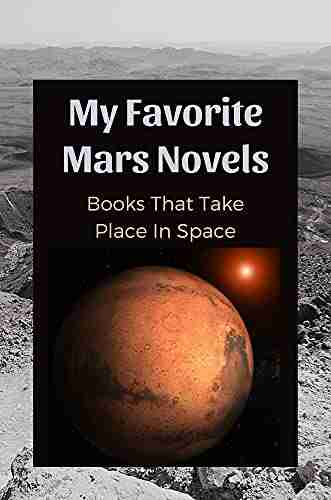

10 Mind-Blowing Events That Take Place In Space
Welcome to the fascinating world of...
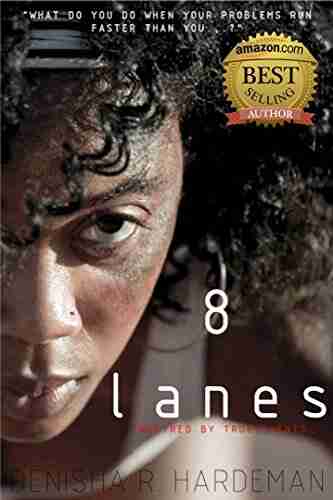

The Astonishing Beauty of Lanes Alexandra Kui: Exploring...
When it comes to capturing the essence of...
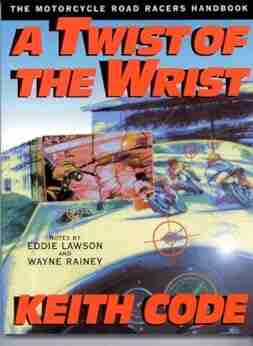

Unlock the Secrets of Riding with a Twist Of The Wrist
Are you a motorcycle...
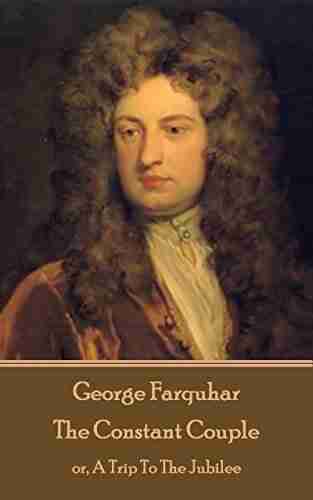

The Ultimate Guide to An Epic Adventure: Our Enchanting...
Are you ready for a truly mesmerizing and...
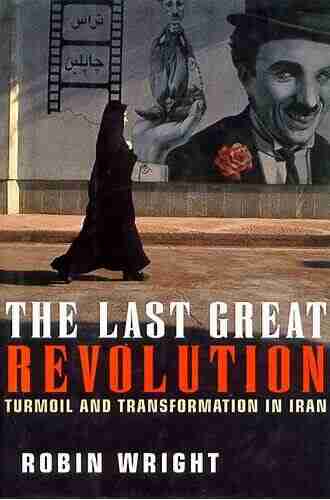

The Last Great Revolution: A Transformation That Shaped...
Throughout history, numerous revolutions have...
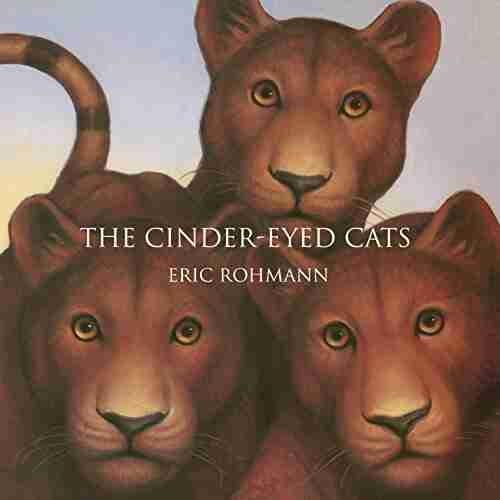

The Cinder Eyed Cats: Uncovering the Mysteries of Eric...
Have you ever come across a book that takes...
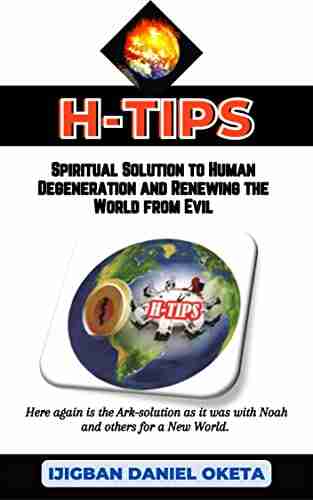

Discover the Ultimate Spiritual Solution to Human...
In today's fast-paced, modern...
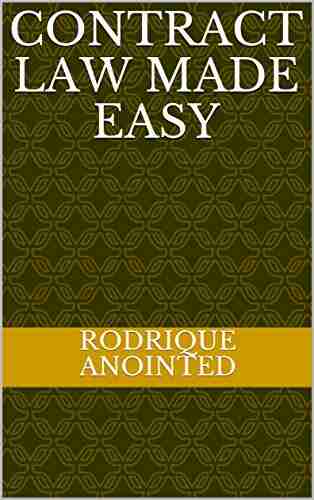

Contract Law Made Easy Vol.: A Comprehensive Guide for...
Are you confused about the intricacies of...
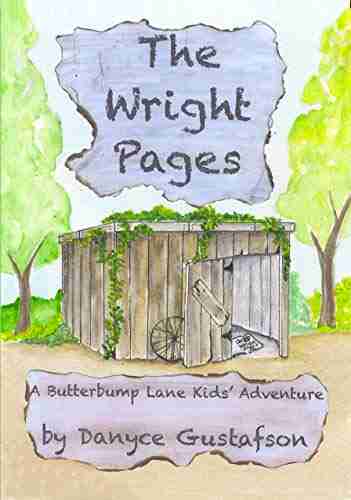

The Wright Pages Butterbump Lane Kids Adventures: An...
In the magical world of...
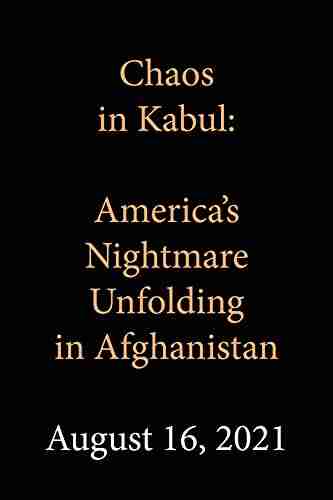

America Nightmare Unfolding In Afghanistan
For more than two decades,...
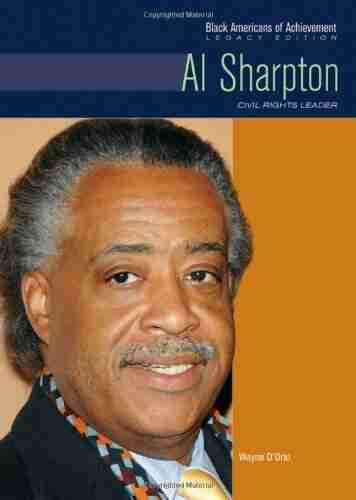

Civil Rights Leader Black Americans Of Achievement
When it comes to the civil...
Light bulbAdvertise smarter! Our strategic ad space ensures maximum exposure. Reserve your spot today!
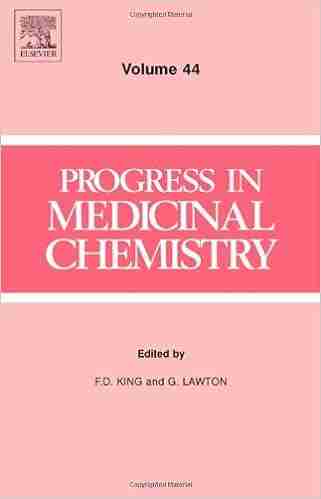

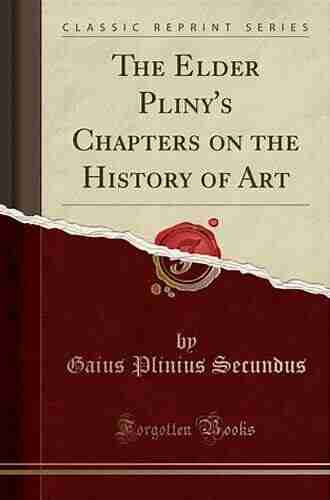

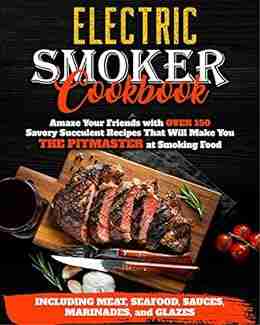

- Victor TurnerFollow ·12.4k
- Garrett BellFollow ·19.2k
- Jorge AmadoFollow ·9.2k
- Eugene PowellFollow ·15.1k
- William PowellFollow ·9.5k
- Carlos DrummondFollow ·10.8k
- Bryson HayesFollow ·2.2k
- Forrest BlairFollow ·9.7k